Much debate surrounds the future of education in the face of Artificial Intelligence. Global and national taskforces are being set up; pedagogic and ethical frameworks are drafted; education is reimagined through the deployment of ‘foundation models’; marketing communications and academic critique equally fill public discourse, draw policy attention, and invite frequent stakeholder convenings. Amidst the noise, excitement and rush, this essay examines the truth that key stakeholders in education should ask for when they discuss and deliberate on the use of AI in education.
Education stakeholders globally have been increasingly concentrating on topics such as the use of generative AI and ‘foundation models’ which promise to adapt and scale education in unprecedented ways. Policymakers and industry leaders alike say that the many promises these technologies hold means that they should be embraced, while teachers and students should be taught as soon as possible the necessary skills and competencies.
AI promises to cut teacher workload, provide support with teaching students, diagnose, plan, recommend learning (and career) pathways, and so on. Indeed, the hope is that such technologies can scale the provision of education to the roughly 260 million children and youth out of school. Yet, AI in education is still mostly discussed in the abstract (which AI, for whom, and why?). Therefore, before going any further, this essay aims to strip down the hyperbole and, just like the child from Andersen’s eponymous fairytale, call out the ‘Emperor’s New Clothes’ and ask for the naked truth.
What is the ‘actually existing AI’?
Stuart Russell and Peter Norvig describe that for AI to work as intended, a long list of complex conditions is required. For AI to benefit education with promises such as automating governance and decision-making, tailoring and adapting learning to one’s unique needs, assessing, generating and recommending the ‘right’ learning pathways, content and assessment and so on, it needs to consider at least the following:
Whether the environment is fully or partially observable. For example, when assessing a student’s performance in class – can we fully observe that?
Whether the environment and actions are discrete or continuous. For instance, are we grading multiple choice answers or, say, creative writing across a time continuum?
Whether the environment contains other variables. For example, a group of pupils work on a project, are we observing all variables during that interaction?
Whether the outcomes of actions as specified by pre-defined ‘rules’or ‘learning styles’. How one learns best according to what subject adds to the complexity of the environment and subjects for which the AI system is expected to function. Various learning styles and conditions shape this environment, some are more predictable and known than others; how well are they embedded in the functionalities of the AI system?
Whether the environment is dynamically changing so that the time to make decisions is tightly constrained or not. Say, pupils conducting a scientific experiment, things may be changing quite quickly and unexpectedly. Is the AI system considering these unpredictable factors?
The length of the horizon over which decision quality is measured according to the objective, which can be very short, of immediate duration or very long. For example, when solving arithmetic problems; developing a research project with periodic evaluations; or long-term – when wanting to measure children’s increased life-chances from studying with an AI application.
Here, we should ask: Which AI proposed in education presently satisfies all these conditions? We can also ask: Which educational environment can claim to be as prescribed as an AI system would need it to be in order for it to function as intended? The answer is no one AI, and no one educational environment.
Before we jump on the AI bandwagon, we are still faced with the numerous conditions and further known and unknown risks to ensure that AI systems achieve their desired goals.
Designing an intelligent tutoring system requires deep understanding of human behaviours, extracting detailed insights from sensory and textual data, and developing adaptive learning processes tailored to individual user needs. But, at what point does this data extraction become ‘project creep’?
The following is from an existing adaptive learning platform. Walkthrough methodology was used to experience its adaptive learning function (among other products as part of wider research evaluating AI-infused educational technologies). It is also an example of automated decision-making – one can argue, an unfair one.

Example of an adaptive learning software used in globally.
First, it is not clear how the system comes about with these problems, and whether it treats all mathematical operations equally. What is the logic from a wrong addition of six-digit numbers for the system to switch to operations with negative and single digits? Second, the system does not provide any meaningful feedback to the student as to how it arrives at these decisions or why the student gave a wrong answer. For learning, feedback is crucial. Third, the student is in a solitary experience with the screen and the platform without necessarily having any social interaction with a teacher or students. Socialisation is vital for learning.
Design decisions for such products are opaque. Many like these are already embedded into practices and supporting decision-making in schools. This kind of ‘AI’ promises education at scale – fifty, hundred, even thousands of students can be placed with this product to study maths. Policymakers see exactly this as attractive because of the economic logics of efficiency such products promise. Studying maths through adaptive learning sounds great to scale. However, efficiency is exactly the opposite of what it takes to learn deeply. AI of this kind can make learning impersonal and dehumanized, devalue the role of teachers, and devalue the purpose of education.
It gets complicated: ‘foundation models’
Even if one claims that such learning environments can be achieved, where the conditions and all variables are fully observable and captured, there lies the additional problem of foundation models. These are types of algorithmic models which are ‘trained on broad data at scale’ and ‘fine-tuned’ or adapted for specific downstream tasks. They certainly entertain us all (ChatGPT can write a poem about my favourite football team emulating Shakespearean voice), but this is far from accepting them in the education domain.
Some researchers argue that ‘foundation models in education could be trained on multiple data sources to learn the capabilities necessary for education’ and then applied ‘in a general-purpose way across a range of tasks and goals such as understanding students, assisting teachers, and generating educational content’.
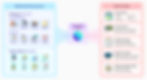
Foundation models in education. Source, Bommasani et al., 2022
Jitendra Malik disagrees with calling AI ‘foundation models’, seeing it as an overstatement because they ‘have only shown their power in’ limited settings. Instead, he prefers to call them ‘castles in the air’ to suggest that they are more like dreamy structures – they might look impressive but they lack depth and evidence. Looking at the image above, these castles suggest extreme homogenisation. To that, Momin Malik says that real-life experiences are devalued.
Similarly, other scholars argue that the common idea of AI envisions a future where huge, standalone systems surpass human abilities in various areas. This view, however, overlooks the social and relational nature of intelligence, which is misleading and harmful. It focuses on mimicking human performance through artificial benchmarks. Lastly, the authors also point that this view also tends to lead to the concentration of power and control over AI – and subsequently where AI are deployed – within a small group of ‘engineering elite’.
The fascination around AI in education should subside, even be contained as Mustafa Suleyman, co-founder of DeepMind proposes, and most of all encourage honesty. What is the actual AI that industry is selling? What are the actual AI products education policymakers wish schools to embrace? When these are stripped down, the naked truth may be neither exciting nor necessary.
This post was first published on Media@LSE blog.